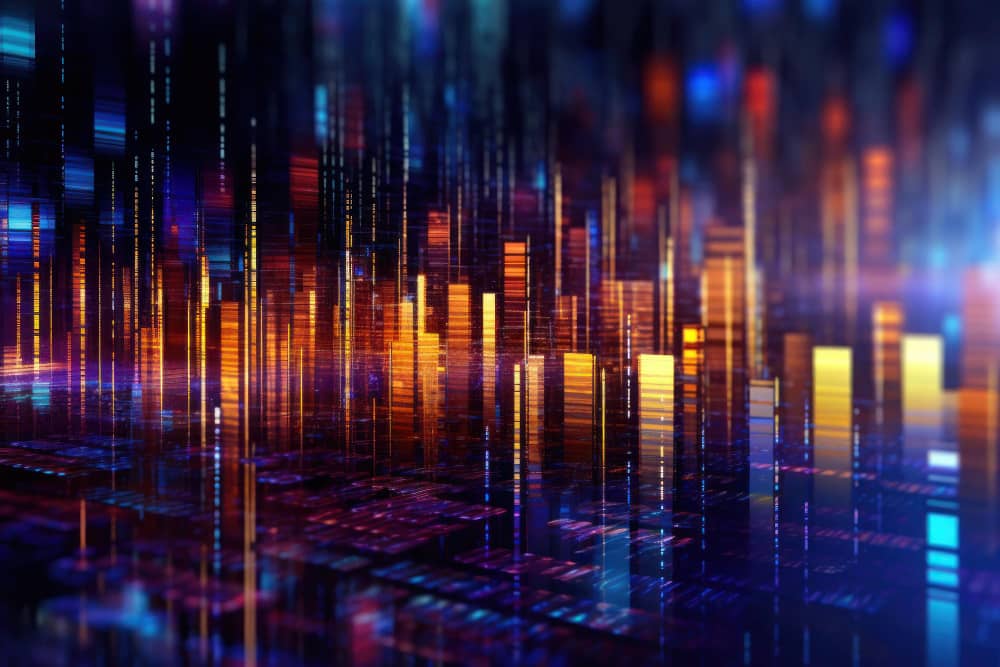
The world of cryptocurrency is a wild, unpredictable beast. Whether you're a seasoned investor or a curious newcomer, the constant ups and downs can be exhilarating or downright daunting. In the midst of this volatility, the promise of AI-generated market predictions offers a beacon of hope for many. But can we trust these predictions? Are they accurate and reliable enough to guide your investment decisions?
In this article, we'll delve into the intricacies of AI-powered foresight in the crypto realm. From the algorithms that power these predictions to their real-world performance, we'll uncover the strengths and pitfalls of relying on artificial intelligence in such a turbulent environment.
"AI is the ultimate tool – but like any tool, it depends on how you use it." – Crypto Analyst
So, whether you're pondering your next big move or just staying informed, let's take a closer look at how these advanced systems stack up against the real-world chaos of the crypto market.
The Mechanics of AI in Crypto Predictions
AI in crypto predictions operates by leveraging complex algorithms and advanced computations to analyze historical market data, on-chain metrics, and various technical indicators. The primary goal? To unearth patterns that aren't readily apparent to the human eye. By processing vast amounts of data at lightning speed, AI algorithms can generate insights that drive predictive models.
One common approach is the use of stochastic modeling, which helps quantify and manage the uncertainty inherent in crypto markets. These models are adept at handling the erratic movements and unpredictable nature of cryptocurrencies. For instance, platforms like CryptoPredictions.ai utilize these AI-driven models to forecast prices across different time horizons – from short-term to long-term – thereby assisting traders in making informed decisions.
Moreover, AI utilizes both supervised and unsupervised learning techniques. In supervised learning, algorithms are trained on labeled historical data to predict future price movements. On the other hand, unsupervised learning allows the system to detect hidden patterns without explicit instruction, often revealing trends that might go unnoticed through conventional analysis.
Another critical aspect is the analysis of on-chain data. By examining transaction histories, wallet holdings, and network activity, AI can generate a more nuanced understanding of market sentiment and investor behavior. This data-driven approach helps in anticipating market shifts more accurately.
In essence, AI doesn't just offer predictions; it elevates the analytical capabilities of traders by converting vast data troves into actionable insights. This blend of cutting-edge technology and meticulous analysis places AI at the forefront of modern crypto trading strategies.
Historical Performance of AI in Crypto Forecasts
One cannot overlook the significance of historical performance when assessing the accuracy of AI in crypto forecasts. Through the lens of historical data, we gain valuable insights into the strengths and weaknesses of AI-driven predictions. By analyzing patterns from the past, AI algorithms aim to provide a logical basis for predicting future market movements.
Several studies have highlighted the proficiency of AI models in capturing broad trends within the cryptocurrency market. For example, breakthroughs in supervised and unsupervised learning have enabled these models to predict price movements with a degree of accuracy that was previously unattainable. These models can decipher complex patterns, identifying signals that human analysts might miss.
Platforms like CryptoPredictions.ai have leveraged this advanced technology to deliver relatively consistent forecast results. They employ historical data sets spanning several years to train their algorithms, fine-tuning them to enhance precision. However, despite these strides, the volatile nature of the crypto market presents notable challenges. Historical performance can only be a piece of the larger puzzle, requiring constant updates and recalibrations of the AI models to adapt to new data and emerging trends.
Therefore, while AI has showcased commendable performance historically, it's important to recognize the dynamic and unpredictable nature of the cryptocurrency landscape. Continuous advancements and rigorous backtesting remain crucial to maintaining and improving the reliability of AI in crypto forecasts.
AI vs Traditional Methods: Which Is More Reliable?
When it comes to predicting the unpredictable world of cryptocurrencies, both AI and traditional methods have their own set of strengths and weaknesses. Traditional methods often rely on historical market trends, expert opinions, and fundamental analyses. These methods, while effective, can struggle to keep pace with the rapid changes and extreme volatility inherent in the crypto market.
On the other hand, AI brings to the table its ability to sift through massive datasets within seconds, identifying patterns and generating insights that might be missed by human analysts. Leveraging tools like neural networks and machine learning algorithms, AI can provide real-time updates and model potential future scenarios. However, the accuracy of these predictions is not always guaranteed. Andrew Ng, a leading figure in machine learning, points out that while AI models have come a long way, they are not infallible. They can still struggle with the unique and often unpredictable factors that impact cryptocurrency markets.
One significant advantage of AI over traditional methods is the use of ensemble methods, which combine multiple AI models to enhance accuracy and robustness. This approach helps mitigate risks associated with overfitting—a common problem where a model performs well on training data but poorly on unseen data. By incorporating ensemble methods, the reliability of AI predictions can be significantly improved. For instance, some AI-driven platforms have shown impressive results by consistently outperforming some traditional benchmarks.
Yet, despite these advancements, it's crucial to remember that AI should be used as one input among many in investment decisions. Laurence Moroney, AI Lead at Google, emphasizes the importance of extracting actionable insights from vast datasets while remaining cautious about the hype surrounding trading bots. A balanced approach, combining the quantitative power of AI with the qualitative insights of human analysts, often yields the best results.
So, which is more reliable? There's no definitive answer. While AI offers advanced tools and methodologies that can enhance the forecasting process, traditional methods provide a depth of understanding and intuition that machines have yet to replicate. The most successful strategies often involve leveraging both AI and traditional methods, creating a more comprehensive and informed approach to navigating the volatile crypto landscape.
The Role of Big Data in AI Market Analysis
Big data plays a pivotal role in enhancing the accuracy and efficiency of AI-driven market analyses. The sheer volume of data generated by the crypto market—ranging from transaction records to social media sentiments—equips AI algorithms with the input required to produce nuanced and informed predictions.
To put this into perspective, consider that every transaction, whether a minuscule trade or a significant shift by a whale investor, contributes to this data pool. When fed into AI models, these datasets become the foundation for predicting future market behaviors. The more data AI has at its disposal, the more precise its predictions can become.
Real-Time Data Processing
One of the most impressive feats of AI in crypto market analysis is its ability to process data in real-time. Unlike traditional methods that might take hours or even days to analyze large datasets, AI can instantly incorporate new information, adjusting its predictions accordingly. This capability is particularly crucial in the volatile crypto market, where timely decision-making can mean the difference between substantial gains and losses.
Social Media Sentiment Analysis
Big data isn't just about numbers. AI algorithms also analyze qualitative data, such as sentiments expressed on social media platforms. For example, a sudden surge in positive tweets about a particular cryptocurrency could signal a bullish trend, while a flood of negative posts might indicate impending downturns. By considering these social cues, AI provides a more comprehensive view of the market landscape.
Data Diversity and Depth
The diversity of data sources is another factor contributing to the success of AI market analysis in the crypto space. From market trades and economic indicators to behavioral analytics and geopolitical events, AI encompasses a wide array of data types. This multi-faceted approach allows for a more sophisticated analysis, capturing nuances that single-source analyses might miss.
In conclusion, big data forms the backbone of AI's ability to predict crypto market trends accurately. As technology continues to evolve, the integration of even more diverse and real-time data sources will likely further refine these predictions, providing traders with ever more reliable insights.
Factors Affecting AI Accuracy in Crypto Predictions
When it comes to predicting crypto market trends, several unique factors can impact the accuracy of AI models. Understanding these factors can help you more effectively utilize AI predictions in your trading strategies.
Market Volatility: The extreme volatility of the cryptocurrency market is one of the most significant challenges. Cryptocurrencies can experience drastic price swings within minutes. While AI can analyze historical data to predict trends, it often struggles to account for sudden market shifts driven by external factors, such as regulatory news or macroeconomic events.
Data Quality and Quantity: AI models rely heavily on vast amounts of high-quality data to generate accurate predictions. Poor quality or insufficient data can lead to misleading forecasts. For instance, any missing or incorrect historical price data can skew results, making the predictions less reliable.
Algorithm Complexity: The sophistication of the AI algorithms also plays a crucial role. Simple algorithms may not capture the intricacies of the market, leading to less accurate predictions. More advanced models, including those using stochastic modeling, can offer better insights but require significant computational resources and expertise to develop and maintain.
External Market Factors: AI struggles to account for unexpected events that have significant impacts on the market. Factors such as regulatory changes, technological advancements, and market sentiment can lead to unpredictable market movements. For example, a sudden regulatory crackdown in a major market can lead to a rapid decline in cryptocurrency prices, a scenario that AI might not predict accurately.
Behavioral Economics: Human behavior in trading often defies logical patterns and can be influenced by emotion, speculation, and hype. These behavioral aspects are challenging for AI to predict accurately, as they don't always follow the historical data trends.
By acknowledging these factors, you can better understand the strengths and limitations of AI in crypto predictions. While AI can provide valuable insights, it is essential to combine these insights with human judgement and market awareness to navigate the volatile crypto landscape effectively.
Limitations of AI in Predicting Crypto Trends
While AI offers a powerful toolset for crypto market predictions, it’s not without its limitations. One of the primary challenges lies in the inherent volatility of the cryptocurrency market. Unlike traditional stocks and commodities, cryptocurrencies can experience rapid and unpredictable price swings due to factors like regulatory news, technological changes, or even social media trends. AI models, especially those reliant on historical data, can struggle to adapt quickly to these abrupt and often irrational market movements.
Moreover, many AI systems in the crypto sphere utilize supervised and unsupervised learning algorithms, each with its own set of constraints. Supervised learning models require large datasets and are highly dependent on the quality of the past data. Poor-quality or noisy data can lead to inaccurate predictions, eroding the reliability of these models. On the other hand, unsupervised learning methods might lack the necessary contextual awareness, making their predictions more speculative.
Another issue is the timeframe suitability for AI predictions. While AI might excel at short-term forecasts where it can quickly adjust to new data, long-term predictions remain a gray area. The longer the forecast horizon, the greater the uncertainty, compounding the effect of unexpected market events that an AI cannot possibly account for in its models.
Another critical limitation stems from the simplifying assumptions made by AI models. For example, many models might overlook psychological factors driving investor behavior, such as fear and greed, which play a significant role in the crypto market. Without accounting for these human elements, AI predictions can miss crucial market movements triggered by collective sentiment shifts.
Finally, regulatory changes pose a significant challenge to AI accuracy. Governments worldwide are still grappling with how to regulate cryptocurrencies, and sudden policy shifts can cause dramatic market fluctuations. Since AI relies on patterns and data, the unpredictability of regulatory interventions can lead to substantial deviations in AI-driven predictions.
Balancing Human Insight and AI Predictions
While AI can process large datasets and identify trends that might take humans much longer to discover, it doesn't replace the nuanced judgment and experience of seasoned investors. For instance, the Chief Investment Officer and their team often use AI tools to supplement their strategies rather than letting the technology dictate actions unilaterally.
Integrating AI with human insight does offer a powerful combination—AI's analytical capabilities paired with human intuition and decision-making prowess can lead to more informed and balanced investment strategies. Successful AI trading models are usually designed to assist in managing risks, minimizing losses, and maximizing gains. However, it's vital to maintain a healthy skepticism and cross-verify what AI predicts with human expertise.
As Laurence Moroney, AI Lead at Google, points out, the true value of AI lies in its ability to extract meaningful information from vast datasets, not in making sole, autonomous trading decisions. Human oversight is essential, particularly in a market as volatile and unpredictable as cryptocurrency.
Moreover, ensemble methods that combine multiple AI models can enhance accuracy and robustness, reducing the risk of overfitting and better capturing the multifaceted nature of the crypto market. This method leverages diverse AI perspectives to produce a more reliable output, which, when coupled with human analysis, can be quite potent.
In everyday investing, you might find AI predictions from services like CryptoPredictions.ai useful for generating ideas or validating your hypotheses. But it’s crucial to use these predictions as one input among many. The volatile crypto landscape requires thorough due diligence and a holistic approach to investment strategies, ensuring AI serves as a helpful assistant rather than the sole decision-maker.
Real-World Applications of AI in Crypto Trading
AI's practical influence in the realm of crypto trading is continually growing, providing traders with a range of innovative tools and strategies. From predictive analytics to automated trading bots, AI is reshaping how market participants navigate the often-turbulent waters of cryptocurrency investments.
Automated Trading Bots: One of the most prevalent applications of AI in crypto trading involves automated trading bots. These bots leverage AI algorithms to execute trades based on predefined criteria and real-time market data, optimizing for speed and accuracy. They operate 24/7, a crucial feature in the always-open crypto market, ensuring that traders can capitalize on opportunities the moment they arise.
Sentiment Analysis: Another significant application is sentiment analysis. AI can sift through massive volumes of social media posts, news articles, and other forms of public discourse to gauge market sentiment. This real-time analysis helps traders understand market mood and adjust their strategies accordingly, potentially giving them an edge over those who rely on traditional news sources.
Predictive Analytics: AI excels in predictive analytics, where it scrutinizes vast datasets to forecast future price movements. By using historical price data, trading volumes, and even external factors like regulatory news, AI can offer short- and long-term predictions that aid traders in making informed decisions. Although not foolproof, these insights can be invaluable in formulating trading strategies.
Risk Management: In addition to predictive capabilities, AI tools are vital for risk management. AI can assess the risk-reward ratio of trades, offer diversification advice, and monitor portfolios for potential red flags. This functionality is particularly beneficial in the volatile crypto market, helping traders mitigate risks and protect their investments.
Overall, the integration of AI into crypto trading platforms offers a blend of efficiency, speed, and sophisticated analysis that is hard to match with traditional methods. As AI technology continues to evolve, its applications in crypto trading are likely to expand, providing even more advanced tools for market participants.
Regulatory Impacts on AI and Crypto Predictions
Regulations play a pivotal role in shaping the landscape of AI-driven crypto predictions. In many jurisdictions, stringent regulations can either stymie or catalyze innovation within this space. For instance, the implementation of the General Data Protection Regulation (GDPR) in Europe has had profound impacts on how data is collected, stored, and processed by AI systems. This becomes especially critical when considering that AI models rely heavily on vast quantities of historical and real-time data to accurately predict market trends.
Moreover, financial regulations targeting cryptocurrencies, such as the U.S. Securities and Exchange Commission’s (SEC) guidelines, add another layer of complexity. These regulations not only aim to safeguard investors but also seek to ensure that AI and crypto prediction services maintain transparency and fairness. Regulatory compliance requires businesses to maintain rigorous documentation and reporting, potentially limiting the agility and experimental nature that often characterizes AI development in the crypto domain.
Another significant factor is the pace of regulatory changes. Cryptocurrency markets evolve rapidly, and keeping up with legal frameworks across various countries can be challenging. This makes it difficult for AI models to adapt swiftly, especially when trained on data sets that might not account for sudden regulatory shifts. As a result, the accuracy of AI predictions can be compromised if the models are not robust enough to incorporate these regulatory changes in real-time.
On the flip side, a well-defined regulatory environment can lend credibility and attract institutional investors to the market, providing a wealth of new data for AI systems to analyze. Legal clarity can also pave the way for wider adoption of AI tools, as investors and stakeholders gain confidence in the market's stability and transparency. The symbiotic relationship between regulation and AI innovation thus becomes a double-edged sword, where both over-regulation and under-regulation can impede the potential benefits of AI in crypto predictions.
As the regulatory landscape continues to evolve, collaboration between policymakers, AI developers, and crypto enthusiasts will be crucial. Open dialogues can help create balanced regulations that encourage innovation while safeguarding stakeholder interests. Keeping an eye on regulatory trends and preparing for compliance can ultimately enhance the reliability and acceptance of AI-driven crypto prediction models, fostering a balanced ecosystem wherein both technological advancements and regulatory frameworks coexist fruitfully.
Conclusion
As the crypto landscape continues to evolve, leveraging AI for market predictions offers both exhilarating possibilities and notable challenges. While AI can process vast amounts of data and uncover hidden patterns far beyond human capabilities, it still grapples with the inherent volatility and unpredictability of crypto markets. Balancing the strengths of AI with human intuition and experience can potentially enhance predictive accuracy. By staying informed about advancements and understanding the limitations, you can better navigate this digital frontier. The future of crypto trading will likely see AI as a crucial ally, but always with room for human insight to interpret and adapt to changing market conditions.