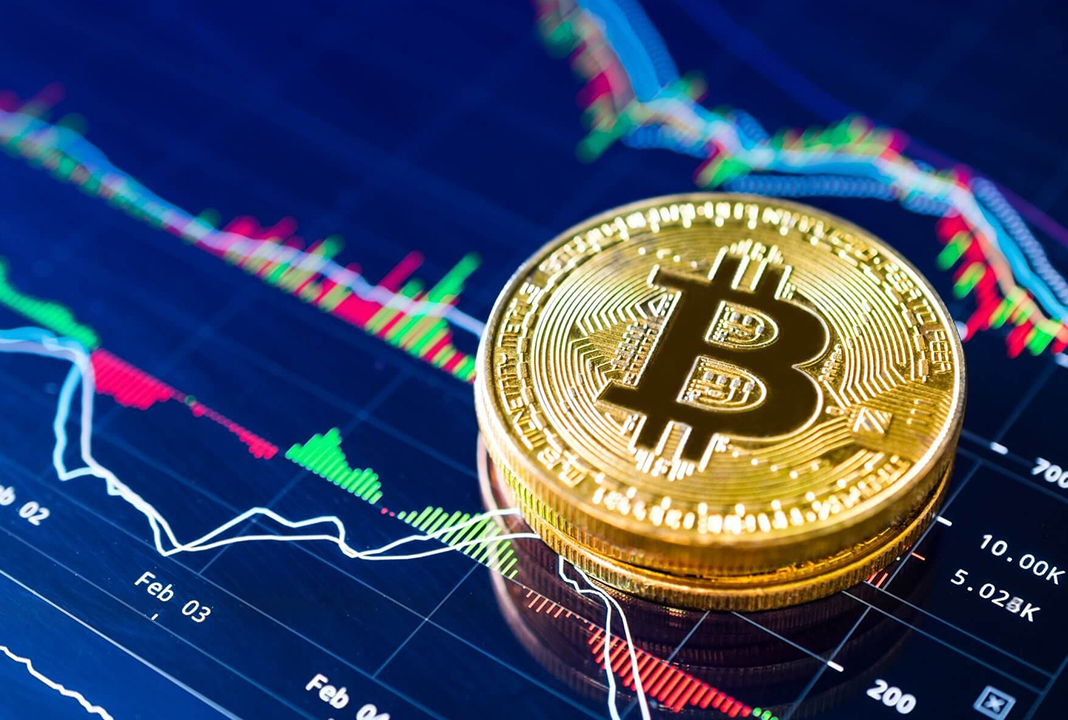
Cryptocurrency trading has taken the financial world by storm, promising high returns and endless possibilities. But navigating the volatile and often confusing crypto markets can be daunting. That's where quantitative crypto trading comes in—leveraging mathematical models and algorithms to make informed trading decisions. Imagine having a consistent, data-driven strategy that can boost your trading performance and reduce risks. Sounds too good to be true? Think again.
In this article, we will delve into the world of quantitative crypto trading, exploring the basics, popular strategies, and the cutting-edge technologies shaping the future of crypto markets. Whether you're a seasoned trader or just dipping your toes in the crypto waters, there's something here for you.
"Quantitative crypto trading isn't just reserved for the elite. With the right knowledge and tools, anyone can harness its power to maximize returns and minimize risks." - An Anonymous Crypto Trader
Stay with us as we break down complex concepts into easily digestible insights, empowering you to make smarter trading decisions. Ready to elevate your crypto trading game? Let's get started!
Introduction to Quantitative Crypto Trading
Quantitative crypto trading relies on mathematical models and statistical techniques to analyze market data and make trading decisions. It's a methodical approach that aims to remove the emotional biases often associated with manual trading. By leveraging historical data and advanced computational tools, quantitative traders can develop strategies designed to exploit market inefficiencies.
One of the key advantages of quantitative trading is its ability to process vast amounts of data quickly and accurately. This allows traders to identify patterns and trends that might be invisible to the human eye. Moreover, it's a discipline that evolves with technology, constantly incorporating new methods such as machine learning and artificial intelligence to improve predictions and results.
As more institutional investors and retail traders enter the crypto space, the demand for sophisticated trading strategies has surged. This has led to the proliferation of quant funds and trading algorithms specifically tailored for the volatility and unique characteristics of crypto markets. By understanding the principles of quantitative crypto trading, you'll be better equipped to navigate this fast-paced and ever-changing landscape.
Understanding the Basics of Quantitative Analysis
Quantitative analysis in crypto trading fundamentally revolves around numeric data and statistical methods to make investment decisions. This approach stands in contrast to qualitative analysis, which might focus more on factors like company management, industry trends, or economic indicators without a rigorous numerical framework. By leveraging quantitative analysis, you aim to model trading opportunities and risks based on concrete, measurable data points.
One of the key elements of quantitative analysis is the use of mathematical models. These models often encompass algorithms that inform high-frequency trading, portfolio optimization, risk assessment, and predictive analytics. Generally, they are designed to capture market inefficiencies and exploit them for profit, often before other investors even realize they exist.
Quantitative analysis typically involves:
- Data Collection: Gathering historical price data, trading volumes, market trends, and other relevant metrics from exchanges and other data sources.
- Data Cleaning: Ensuring the data is accurate, consistent, and free from errors that could skew analysis results.
- Model Selection: Choosing an appropriate statistical or machine learning model that fits the trading strategy you're testing.
- Parameter Optimization: Fine-tuning the model's parameters to enhance its predictive accuracy and profitability.
- Backtesting: Applying the model to historical data to see how it would have performed in the past, thus gauging its potential future success.
In essence, the purpose of quantitative analysis in crypto trading is to remove as much of the guesswork as possible. By relying on empirical evidence and statistical rigor, you can develop a more robust, data-driven approach to trading. This can help you identify opportunities that might be invisible through conventional analysis techniques and manage risk more effectively.
As advanced as it sounds, quantitative analysis isn't exclusive to professional prop traders and institutional investors. With the advent of sophisticated trading platforms and accessible computational tools, retail traders can also leverage quantitative strategies. Nevertheless, it is crucial to have a sound understanding of both the underlying mathematical concepts and market dynamics to effectively use quantitative analysis in crypto trading.
The Importance of Data in Quantitative Trading
Data is the lifeblood of quantitative trading. In crypto markets, where uncertainty is a constant companion, having access to accurate and comprehensive data can be the difference between success and failure. But it's not just about having data – it's about having the right data and knowing how to use it effectively.
First, let's talk about the types of data you need. Historical price data forms the cornerstone of any quantitative strategy. This data provides insights into how different crypto assets have behaved over time, revealing trends, patterns, and potential trading signals. Additionally, volume data offers a lens into the market’s liquidity and the intensity of trading activity, which can be crucial for executing trades without significant price impact.
Another critical data type is order book data. This data helps you understand the market depth and the placement of buy and sell orders at different price levels. Having a clear view of the order book can inform your decisions about when to enter or exit a position and at what price.
Moreover, alternative data like social media sentiment, blockchain activity, and macroeconomic indicators also play a significant role. In the fast-paced world of crypto, market sentiment can shift rapidly based on news, tweets, or even rumors. By incorporating alternative data, you can gauge the market mood and anticipate potential movements that aren't immediately obvious from price data alone.
The ability to analyze and interpret this data effectively is what sets successful quantitative traders apart. Analytical tools such as statistical software, machine learning algorithms, and custom-built models are indispensable. They help you to process and make sense of extensive datasets, identifying patterns and signals that would be impossible to spot manually.
Furthermore, data integrity and reliability are paramount. Ensuring that your data sources are accurate and up to date is critical. Inaccurate or outdated data can lead to faulty analyses and misguided trading decisions. Employing quality control measures, like verifying data from multiple sources and regularly updating your datasets, can help maintain the integrity of your analyses.
Ultimately, the strategic use of data allows you to develop, validate, and hone your trading strategies. By leveraging high-quality data and sophisticated analytical tools, you can gain a competitive edge in the volatile and ever-evolving world of crypto trading.
Popular Quantitative Crypto Trading Strategies
When it comes to crafting a profitable strategy, understanding various approaches can make a significant difference. Here, we’ll delve into some of the cornerstone strategies frequently leveraged by quantitative crypto traders.
Statistical Arbitrage
Statistical arbitrage, often referred to as "stat arb," involves exploiting price discrepancies between correlated crypto assets. By employing statistical models, traders can identify and profit from short-term inefficiencies in the market.
- Market Neutrality: This strategy aims to be market-neutral, minimizing exposure to market movements while capitalizing on price differentials.
- Pairs Trading: A popular approach within stat arb, pairs trading involves matching a long position in one crypto with a short position in another, balancing gains and losses.
Algorithmic Trading
Algorithmic trading uses automated software to execute trades based on predefined criteria. This method can process large volumes of data swiftly, execute trades at optimal prices, and operate around the clock.
- High-Frequency Trading (HFT): HFT seeks to execute a large number of orders at extremely high speeds, often capitalizing on tiny price fluctuations.
- Market Making: Involves placing both buy and sell orders to profit from the bid-ask spread, improving market liquidity in the process.
Sentiment Analysis
This strategy analyzes data from social media, news sources, and other public platforms to gauge market sentiment. By quantifying the emotional tone conveyed in public discourse, traders can make informed decisions about buying or selling assets.
- Natural Language Processing (NLP): Tools leveraging NLP can process vast amounts of text data to detect trends and sentiment changes in real-time.
- Predictive Analysis: Combining sentiment data with historical price information to forecast future price movements.
These strategies rely heavily on robust backtesting and rigorous data analysis, crucial components we will discuss further in upcoming sections. Exploring and understanding these different strategies can provide you with the tools needed to navigate the volatile world of crypto trading more effectively.
Mean Reversion Strategies
At its core, mean reversion theory posits that asset prices and historical returns will eventually return to their long-term average or mean. Applying this theory to crypto trading involves identifying assets that have deviated significantly from their historical averages and predicting that they will revert to those levels over time.
Imagine a cryptocurrency that has an average price of $100 over the past year but suddenly drops to $70. A mean reversion strategy would suggest that this drop is temporary and the price will eventually climb back to its average of $100. Thus, a trader might buy the cryptocurrency at $70 in anticipation of its return to $100.
The success of mean reversion strategies hinges on the identification of suitable entry and exit points. Traders utilize various tools and indicators to determine these points, including:
- Moving Averages: These help in identifying the average value of a crypto asset over a specific time period, providing context for detecting deviations.
- Bollinger Bands: This volatility indicator shows the upper and lower limits of an asset's price range, offering cues on potential overbought or oversold conditions.
- Relative Strength Index (RSI): This momentum oscillator measures the speed and change of price movements, signaling overbought or oversold states.
Furthermore, mean reversion can be implemented within multiple timeframes, ranging from intra-day trading to longer-term investments. Day traders might look for opportunities where prices deviate from short-term averages, while long-term investors might focus on broader market corrections.
However, it's vital to recognize the limitations and risks associated with mean reversion strategies. The fundamental assumption is that past prices will predict future movements, which may not always hold true, especially in the highly volatile crypto markets. Therefore, it is paramount to combine this strategy with robust risk management practices and continually monitor market conditions.
In essence, mean reversion strategies can be a powerful tool in a trader's arsenal when approached with careful analysis and a disciplined trading plan.
Momentum Trading Strategies
Momentum trading strategies are exciting approaches that capitalize on the strength of existing market trends. At their core, these strategies involve buying cryptocurrencies that have shown an upward price movement or shorting those in a downtrend. The basic idea is to ride the momentum until it shows signs of reversing.
One popular technique within momentum trading is breakout trading. Here, you identify price levels that an asset has consistently struggled to surpass. Once the price breaks through these levels, it often continues to move in that direction for some time. By entering trades at these breakout points, you can potentially capture significant gains as momentum drives the price further.
Another approach is moving average crossovers. This involves using two different moving averages (MAs), typically a shorter and a longer period MA. A buy signal is generated when the shorter period MA crosses above the longer one, indicating upward momentum. Similarly, a sell signal occurs when the shorter MA crosses below the longer one, reflecting downward momentum.
It's crucial to continuously analyze momentum by measuring indicators such as the Relative Strength Index (RSI) or the Moving Average Convergence Divergence (MACD). These tools help assess the strength and potential sustainability of a trend, offering valuable insights to fine-tune your entries and exits.
As with any strategy, risk management remains paramount. Utilizing stop-loss orders and having a well-devised exit plan can safeguard your capital from sudden market reversals. Furthermore, consider implementing a trailing stop loss, which adjusts your stop-loss level as the trade moves in your favor. This technique locks in profits while protecting against downside risks.
In summary, trading momentum strategies offer substantial opportunities by harnessing the force of market trends. However, it's essential to combine technical indicators with robust risk management practices to navigate this dynamic trading environment successfully.
Arbitrage Opportunities in Crypto Markets
Arbitrage opportunities in crypto markets leverage price discrepancies for the same asset across different exchanges. Simply put, it’s buying low on one exchange and selling high on another. These opportunities arise due to the highly fragmented nature of cryptocurrency exchanges, varied trading volumes, and differences in liquidity.
One common type of arbitrage strategy is spatial arbitrage. This involves the simultaneous purchase and sale of an asset in different markets to capitalize on the price differences. For instance, if Bitcoin is cheaper on Exchange A than on Exchange B, a trader can buy Bitcoin on Exchange A and sell it on Exchange B, pocketing the difference as profit.
Another popular method is triangular arbitrage. This entails taking advantage of the price differences between three different cryptocurrencies. For example, starting with Bitcoin (BTC), you might find that the exchange rates among Litecoin (LTC) and Ripple (XRP) offer a potential profit. You’d convert BTC to LTC, LTC to XRP, and finally XRP back to BTC, ideally increasing your BTC holdings in the process.
However, arbitrage is not without its challenges. Trades must be executed rapidly to capitalize on these opportunities before they disappear, often within mere seconds. Additionally, transaction fees, withdrawal fees, and timing issues can eat into profits. Therefore, reliable infrastructure, liquidity, and precise algorithms are key to successful arbitrage trading, making it predominantly a game for high-frequency traders and sophisticated bots rather than individual retail investors.
High volatility in the crypto markets further contributes to arbitrage potential but also adds to the risk.
- Pros: Potential for risk-free profit, exploiting price inefficiencies.
- Cons: Requires quick execution, high fees, and possible market movement risks.
In essence, while arbitrage can seem like a golden opportunity, it demands diligence, advanced tools, and a deep understanding of the market mechanics to be profitable consistently.
Machine Learning and AI in Crypto Trading
Machine learning and artificial intelligence (AI) have dramatically changed the landscape of crypto trading, offering sophisticated tools to uncover patterns and generate profitable strategies. At its core, machine learning involves training algorithms on historical data to recognize patterns and make predictions without explicit programming. In the fluid and often volatile crypto markets, this ability to swiftly analyze and adapt to new data provides a competitive edge.
One of the prominent applications of machine learning in crypto trading is predictive modeling. By harnessing vast amounts of historical data and machine learning algorithms, traders can create models that forecast future price movements based on past trends and market signals. This predictive capability allows traders to make more informed decisions, enhancing the potential for profitable trades.
Another vital application is in the realm of portfolio management. AI can optimize asset allocation by continuously analyzing market conditions and adjusting the portfolio to maintain the desired risk-reward balance. This dynamic adjustment can help traders maximize returns while minimizing risk, a crucial aspect of effective trading strategy.
Furthermore, natural language processing (NLP), a subset of AI, is increasingly utilized to gauge market sentiment. By analyzing news articles, social media feeds, and other textual data, NLP algorithms can identify prevailing market moods and sentiments, providing traders with actionable insights. For example, a surge in positive sentiment regarding a particular cryptocurrency might indicate a good buying opportunity, while negative sentiment could signal a potential decline.
However, while machine learning and AI offer powerful tools for crypto trading, they also come with inherent challenges. Data quality and availability, computational costs, and the complexity of model tuning can pose significant hurdles. Traders must continuously monitor and adjust their algorithms to ensure they remain effective in changing market conditions.
In summary, integrating machine learning and AI into crypto trading strategies opens up new possibilities for predictive accuracy and automated decision-making. By leveraging these advanced technologies, traders can navigate the complexities of the crypto market more effectively, potentially enhancing trading performance.
Backtesting and Simulation Techniques
One of the cornerstone practices in developing robust quantitative crypto trading strategies is backtesting. By applying a proposed trading strategy to historical market data, traders can simulate the potential performance of their approach without risking actual capital.
Why is backtesting essential?
Backtesting allows traders to identify both the strengths and weaknesses of their strategies. You can fine-tune parameters and gain confidence before applying them in real-time scenarios. Essentially, it provides empirical evidence to support or refute the effectiveness of a trading strategy.
Steps for Effective Backtesting
- Data Collection: Gather high-quality historical data relevant to the crypto assets you aim to trade. Ensure the data is clean and free of significant anomalies.
- Define Strategy Rules: Clearly articulate the rules and conditions that govern your trading strategy. This includes entry and exit points, risk management protocols, and any other criteria.
- Simulate Trades: Apply the strategy rules to the historical data and simulate the trades. Record each simulated trade's results, including wins, losses, and overall profitability.
- Analyze Results: Examine the simulation outcomes critically. Look for metrics such as profitability, drawdowns, win/loss ratios, and other performance indicators.
- Optimize and Refine: Based on the analysis, tweak and optimize your strategy. Retest to see if the adjustments lead to improved outcomes without overfitting to the historical data.
Simulation Techniques
In addition to basic backtesting, more advanced simulation techniques can add depth to your strategy validation process:
- Monte Carlo Simulations: These involve running the strategy through thousands of random market scenarios to gauge its robustness under different conditions.
- Walk-Forward Optimization: This method segments historical data into multiple in-sample and out-of-sample periods to continuously recalibrate and test strategies.
- Agent-Based Modeling: Create virtual "agents" that simulate different market participants and test how your strategy performs against them to assess adaptability.
By utilizing comprehensive backtesting and simulation techniques, you can effectively validate the viability of your trading strategies, mitigate potential risks, and enhance your confidence before executing them in live markets. This methodical approach is crucial for achieving sustained success in the world of quantitative crypto trading.
Risk Management in Quantitative Trading
Effective risk management is the cornerstone of any successful quantitative trading strategy. Without it, even the most sophisticated models can quickly lead to substantial losses. So, what exactly does risk management entail in the realm of quantitative crypto trading?
Diversification: One of the fundamental principles is diversification. By spreading investments across different cryptocurrencies and trading strategies, you minimize the impact of poor performance in any single asset or model. This approach helps in balancing out risk and reward.
Position Sizing: Another vital aspect is position sizing. This involves determining the amount of capital to allocate to each trade. Using mathematical models, traders can optimize position sizes to ensure that no single trade can significantly impact the overall portfolio.
Stop-Loss Orders: Implementing stop-loss orders is another key tactic. These orders automatically sell a position when it hits a certain price level, thereby limiting potential losses. By setting predefined exit points, you can protect your capital from drastic market swings.
Risk-Reward Ratio: It’s also crucial to consider the risk-reward ratio. This ratio evaluates the potential profit of a trade against the potential loss. A favorable risk-reward ratio ensures that the potential gains justify the risks taken, enhancing overall profitability in the long run.
Volatility Analysis: Understanding and analyzing market volatility is indispensable. Volatility can significantly affect trading outcomes, so using statistical measures, like standard deviation and the VIX index, can help in predicting and preparing for market fluctuations.
Continuous Monitoring and Adjustments: Lastly, continuous monitoring and adjustments are imperative. Markets evolve, and so should your strategies. By regularly reviewing performance and making necessary adjustments, you can stay ahead and mitigate risks effectively.
In summary, risk management in quantitative crypto trading involves a multifaceted approach. From diversification and position sizing to stop-loss orders and volatility analysis, each element plays a crucial role in safeguarding your investments. By employing these techniques, you not only protect your capital but also enhance the potential for consistent, long-term success.
Challenges and Limitations of Quantitative Crypto Trading
Quantitative crypto trading, while promising, comes with its own unique set of challenges and limitations. One of the primary challenges is the inherent volatility of the crypto market. Cryptocurrencies are known for their dramatic price swings, which can happen in a matter of minutes. This extreme volatility can render some quantitative models less effective or even obsolete within a short period.
Another significant limitation is data reliability. The accuracy and availability of historical data are crucial for developing and backtesting trading strategies. However, the crypto market is still relatively young, and the data available may not be as extensive or consistent as that for traditional assets. Furthermore, different exchanges might provide varying data, adding another layer of complexity.
Backtesting itself presents limitations. One notable issue is the inability to know intra-candle price movements. This gap can affect the accuracy of simulations, although using lower (faster) timeframes can somewhat mitigate this problem. Platforms like Freqtrade offer backtesting functionalities, but they too have historic limits that might inflate trading limits based on past prices, leading to unrealistic expectations.
Exchange-specific issues also pose a challenge. Many exchanges impose precision limits on price and amounts traded. These constraints can lead to rounding or truncating values, which can impact the execution of trades and overall strategy performance. Additionally, liquidity issues on smaller exchanges can result in slippage, where trades are executed at different prices than expected, thereby affecting returns.
Despite these obstacles, quantitative traders can take proactive steps to minimize risks. By going through 30 to 50 trades during backtesting, traders can improve their pattern recognition skills and gain a deeper understanding of price behavior. Backtesting allows traders to identify the strengths and weaknesses of their strategies, refine parameters, and build confidence. However, it's essential to remember that backtesting cannot completely predict future performance due to market unpredictability and the ever-changing dynamics of crypto trading.
In conclusion, while the challenges and limitations in quantitative crypto trading are numerous, they are not insurmountable. Knowledge, preparation, and continuous adaptation are key to navigating the complex landscape successfully.
Benefits of Using Quantitative Strategies for Crypto Trading
One of the significant advantages of employing quantitative strategies in crypto trading is the elimination of emotional bias. As human traders, emotions like fear and greed can often cloud judgement, leading to suboptimal decisions. Quantitative strategies, being rooted in mathematical models and statistical analysis, ensure that trading decisions are based on objective data rather than subjective feelings.
Additionally, quantitative trading strategies allow for greater consistency. By adhering to a predefined set of rules, these strategies ensure that trades are executed uniformly, leading to a more disciplined approach to the market. This consistency can be especially beneficial in the volatile world of cryptocurrency, where market conditions can change rapidly.
Moreover, quantitative strategies can process and analyze vast amounts of data far more efficiently than a human could. This ability to handle large datasets means that these strategies can identify patterns and opportunities that might be missed by manual analysis. This edge is crucial in markets like cryptocurrency, where timely and accurate information can make all the difference.
Another key benefit is the customization and scalability that quantitative strategies offer. Traders can tailor these strategies to fit their specific risk tolerance, investment goals, and market outlook. Furthermore, once a quantitative strategy is developed and backtested successfully, it can be scaled up to manage larger sums of capital, unlike manual trading approaches which may face limitations as the capital grows.
Finally, quantitative strategies provide a clear framework for performance evaluation. By utilizing backtesting and simulation techniques, traders can validate their strategies before risking actual capital. This empirical evidence is invaluable, as it supports data-driven decision-making and helps in identifying the strengths and weaknesses of a trading approach. Consequently, this can increase a trader's confidence in their strategy, making them better prepared for live trading.
In conclusion, the use of quantitative strategies in crypto trading offers multiple benefits, including eliminating emotional bias, ensuring consistency, processing large volumes of data, offering customization and scalability, and providing a solid framework for performance evaluation. These advantages make quantitative strategies an attractive option for traders looking to enhance their trading performance and achieve their financial objectives.
Conclusion and Key Takeaways
Quantitative crypto trading is a multifaceted field that leverages statistical methods, algorithmic models, and technological advancements to execute trades more effectively. The various strategies we have discussed, from statistical arbitrage to momentum trading, each offer unique advantages and cater to different trading goals and risk appetites. One of the defining traits of quantitative trading is its reliance on data-driven decisions and computational power, which can help traders capture market inefficiencies and gain a competitive edge.
Incorporating these strategies involves significant groundwork in data collection, backtesting, and constant refinement. Effective risk management practices are essential to mitigate losses and protect your investments. Tools like machine learning and AI further enhance the accuracy and efficiency of trading algorithms, providing deeper insights and better predictive capabilities.
However, quantitative trading is not without its challenges. Market conditions are constantly evolving, and the complexity of developing and maintaining sophisticated models can be daunting. Despite these challenges, the potential benefits make it a compelling approach for those willing to invest the time and resources.
Here are the key takeaways:
- Quantitative trading strategies utilize data and statistical models for more informed trading decisions.
- Diverse strategies like statistical arbitrage, algorithmic trading, and sentiment analysis cater to various market conditions.
- Data quality, backtesting, and simulation techniques are crucial for building robust trading models.
- Risk management is paramount to safeguard against market volatility and unforeseen risks.
- Machine learning and AI are increasingly vital tools in enhancing trading strategy accuracy and efficiency.
- Constant monitoring and adaptability are necessary to maintain the relevance and performance of trading strategies.
By harnessing the power of quantitative trading, you can navigate the complex crypto markets with greater confidence and precision. Equip yourself with the right tools and knowledge, and you're well on your way to achieving your trading goals.